Operational leaders within the Oncology service line are fully aware of exactly how difficult it is to balance the multiple tradeoffs that must be made in order to schedule infusion services efficiently. Patients would like to have a consistent schedule for each week of their 8-week infusion regimen; nurses would like a predictable schedule to eat lunch and to end their shift on time each day; the clinical staff would like to ensure that the infusion schedule has the flexibility to accommodate a last-minute add-on or a new, emergent case; the Finance and Administrative leadership would like the chairs and nursing staff in the infusion center to have a high (but manageable) level of utilization.
One might assume that this would be a simple scheduling problem. All that is needed is to ensure that a chair is available for each patient at the scheduled time of their appointment and when you run out of available chairs, you don’t offer up appointments until a chair frees up from a patient who has completed their infusion treatment. The talented nursing staff ought to be able to manage the workload if the chairs are clustered into pods and each pod has the right number of nurses with the appropriate skill set. Nothing could be further from the truth!
The demand side of the equation is volatile and difficult to predict. Since a typical regimen runs for 6-8 weeks, the patient population coming to the infusion center is continuously evolving. Each patient has a unique treatment protocol that requires a built-to-order formula of medications and a specific duration for their infusion. Patients often need to see their oncologist in the clinic prior to each infusion in order to ensure that they are healthy enough to undergo infusion – each oncologist has a clinic schedule for a different set of days each week. Finally, the timing of patient arrivals is also hard to predict – for no fault of theirs, the clinic visit may be running late or their lab tests take longer to be processed than anyone had anticipated.
The supply side of the equation is constrained, difficult to predict and heavily interdependent. There are a finite number of chairs that have a fixed number of operational hours in the day. There are only so many infusion pumps that are ready for deployment and a fixed number of pharmacy hoods that can be used to formulate the medications needed by each patient. The nursing roster has a specific number of nurses and, on any given day, one or more nurses may call in sick. In order for an infusion treatment to proceed, all four elements of supply – the chair, the pump, the drugs and the nurse have to be available and ready when the patient arrives.
This explains why matching a volatile demand signal with a constrained, interdependent supply signal is so difficult – especially given that both signals are hard to predict with a high level of precision. Against, this backdrop is the reality that nearly all infusion appointments are scheduled by two people (patient/scheduler, patient/provider or provider/scheduler) looking at a calendar and simply deciding that John Doe should be given the 9:30 a.m. slot on the coming Wednesday. How could this possibly work since that decision did not involve a detailed analysis of either the demand side or the supply side of that equation? Being surprised that the infusion center invariably ends up in a “rush-hour” effect at midday and long waiting times for patients is analogous to being surprised that your phone hits the ground every single time you drop it. Gravity is always present and so is the mathematical complexity of scheduling infusion appointments. Ignoring it doesn’t make it go away !!
Infusion scheduling could be thought of as a challenging game of Tetris. Each appointment has a certain duration which is represented by the length of the Tetris block. The trick is to organize the Tetris blocks in a manner that minimizes the “holes” in the Swiss cheese and allows the center to operate efficiently.
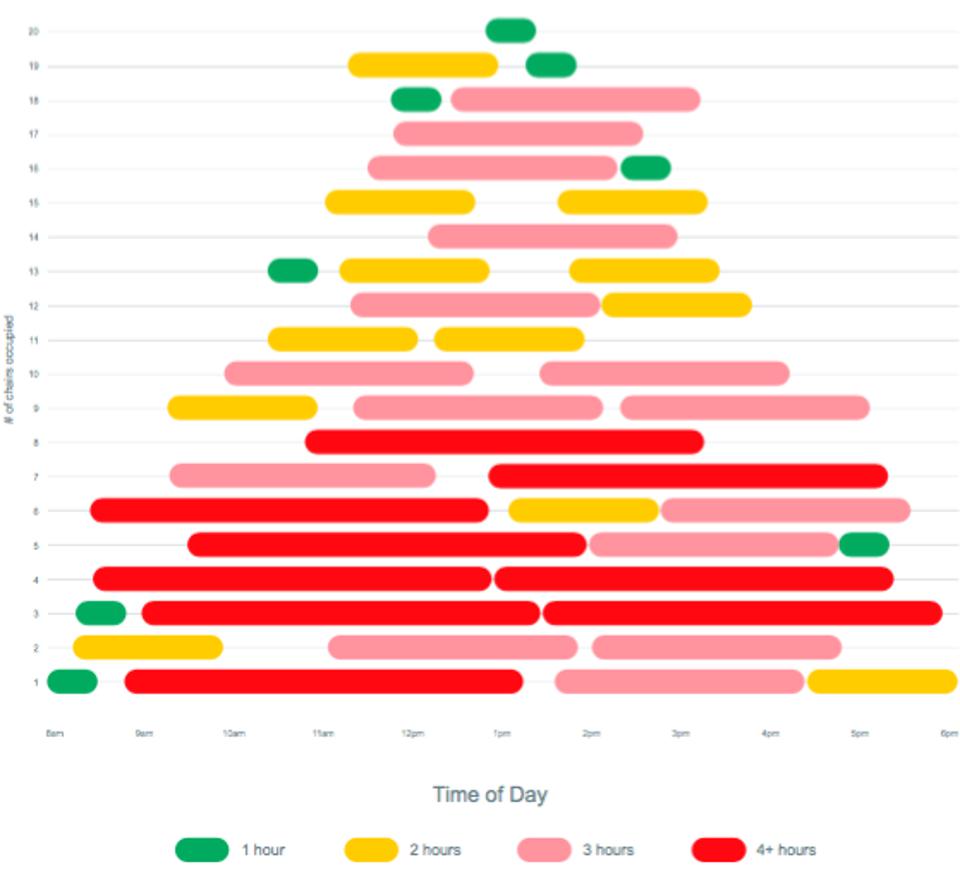
Consistently winning the game of Infusion Scheduling Tetris requires superb, daily execution against the following steps:
- Forecast the volume of patients for each day of the week. Infusion is a “day of week” game – since each oncologist practices on certain days of the week. Mining historical data from the most recent 6-12 months can enable the creation of accurate forecasting models that take into account the various factors such as seasonality and the changes in the number of oncologists on the staff.
- Forecast the duration mix for each day of the week. Again, the historical data is a goldmine of information in order to be able to accurately estimate the number of treatments that will have durations of 1-hour, 2-hour, 3-hour and so on.
- Adjust for the reality of the accuracy (or inaccuracy) of duration estimation in your infusion center. Schedulers have built “cheat-sheets” over a period of years to translate the orders received from the oncologist into a specific treatment regimen involving a certain duration. Comparing the bell curve of the actual treatment duration against the expected duration is hugely informative – it enables adjusting the size of the Tetris block to match your reality. If the bell curve for each duration is tightly spread around the correct length, retain the size of the Tetris block. If the bell curve for a specific duration demonstrates that it consistently runs longer than planned, then expand the contract block. Or, shrink it if the bell curve indicates that the appointment consistently gets completed in a shorter-than-expected duration.
- Build constraint-based optimization algorithms. There are many constraints that need to be factored into the optimization algorithm for each of the four supply elements – the chair, the pump, drugs and the nurse. Chairs are available for certain hours of the day and need to be thoroughly cleaned between patients. Some chairs may need to be set aside for “fast track” or injections/port draws. There are a finite number of pumps which need to be cleaned and sterilized between patients. Drugs often have components with very short shelf-lives (e.g., a few hours) and are fully tailored to a specific patient. Therefore, they cannot be pre-mixed in advance and can only start to be mixed after the patient has checked in and is authorized for treatment. Nursing constraints include the number of nurses and the skillset of the nurse (e.g., some nurses are more trained to work with patients with bone marrow transplant patients for example). They also include the safety protocols in place at each center that govern how many new treatments can be started by a specific nurse each hour or the upper limit on the number of patients that can be simultaneously cared for by a specific nurse.
- Build optimized templates. The output of the prior steps is an optimized template with prescriptive guidance on the ideal options for scheduling an upcoming appointment based on the duration of the treatment and the available slots for that duration on the specific day.
- Run each day based on the actual reality. Infusion scheduling is not a “set-and-forget” activity. While optimized templates can help steer patients into the right slot based on their duration and all of the constraints described above, the actual reality on any specific day might be quite different. This could be due to the volumes or the duration mix deviating from the forecast level. It could also be due to certain aspects of the operation such as the Lab, Pharmacy or the Clinics running later than expected. Over time, the templates will naturally drift from the optimum and need to be tuned through algorithmic learning that identifies the root causes for the drift and automatically adjusts the underlying models.
Optimizing infusion scheduling has an enormous impact – it will enable your infusion center to complete 10-15% more treatments within the existing hours of operation and staffing levels. This is important in providing improved access to new patients who need to begin their infusion regimen. Optimization also helps reduce the wait time for patients which is a critical element for patient satisfaction. Although the quality of the cancer treatment is the biggest driver of patient satisfaction, the single biggest source of patient dissatisfaction is excessive wait time. Finally, staff satisfaction will also improve – providing infusion services to patients is an incredibly stressful job for nurses who need to make sure that they are not so harried that they make a mistake since the consequences can be quite severe.
Mastering the age old game of Tetris is therefore a noble pursuit in the quest to provide superior services to cancer patients who need all of the help and support that they can get to overcome their challenging situation.
We get into additional detail of optimizing the operational performance of infusion centers in Chapter 8 of the book “Better HealthCare Through Math.” You can also reach us at info@leantaas.com for more information on how hundreds of infusion centers that are part of the leading cancer institutions in the country have deployed this approach.
This article originally appeared on Forbes’ Marketplace Blog.